Was Amazon Go the future of retail or a billion-dollar experiment that proved AI alone isn’t enough?
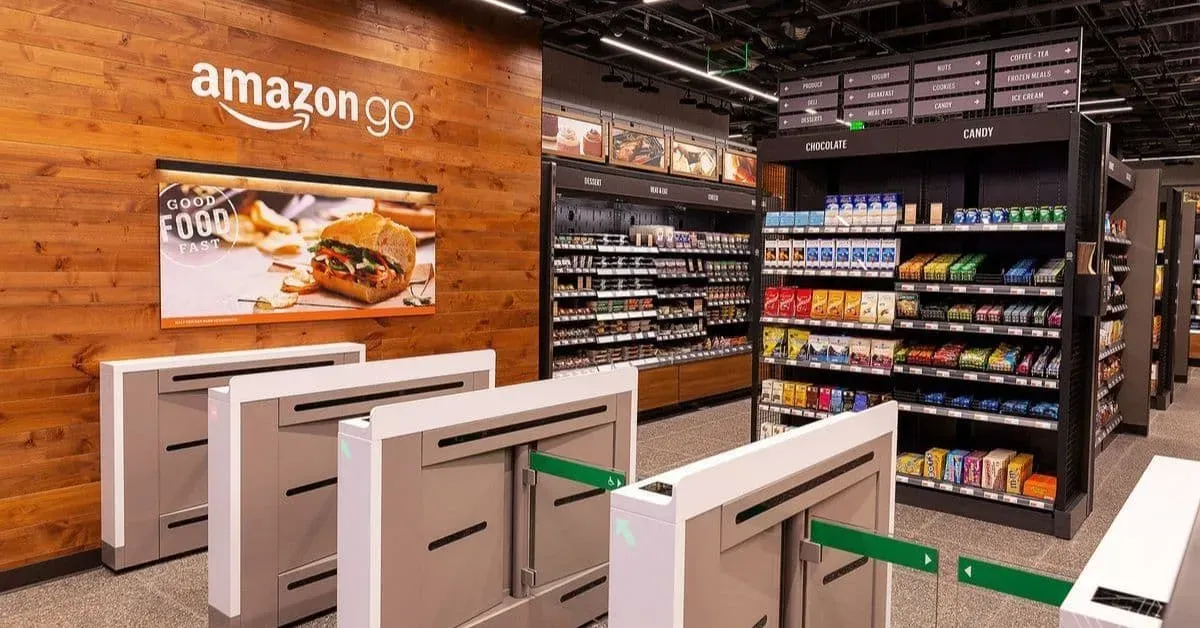
Amazon Go set out to eliminate checkout lines, relying on AI and automation to track purchases. But today, the model has been scaled back. Was it a failure or a necessary step toward the future of frictionless retail?
🛠 How Amazon Go's "Just Walk Out" Technology Worked
Amazon Go's Just Walk Out (JWO) system combined computer vision, AI, and sensor fusion to enable a cashierless shopping experience.
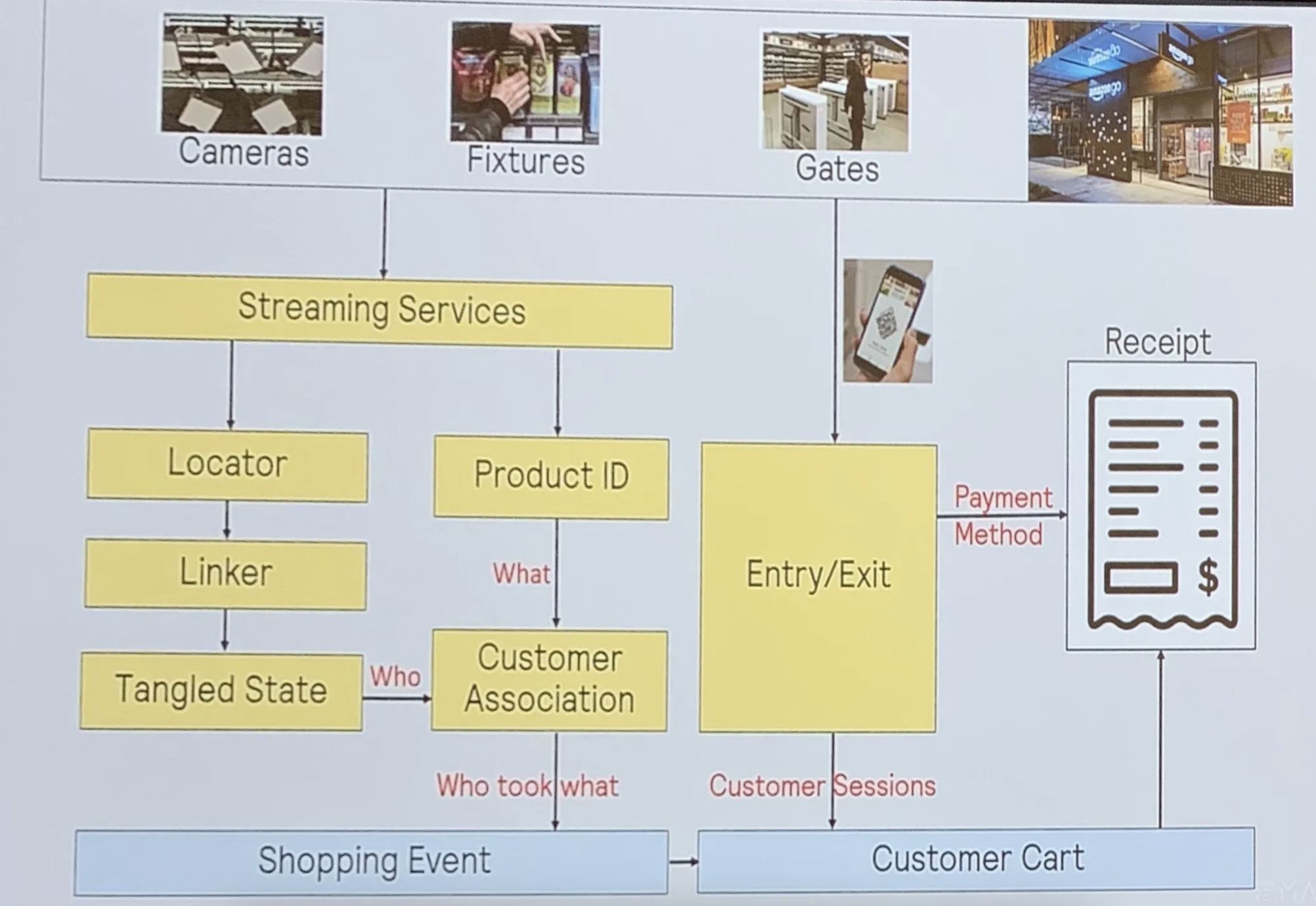
- Step 1: Entry & Identification → Customers scan their Amazon app at the gate.
- Step 2: AI-Powered Tracking → Cameras & sensors detect product movements.
- Step 3: Smart Cart Management → Items added/removed from the virtual cart in real-time.
- Step 4: Auto Checkout & Payment → Customers walk out, and their Amazon account is charged.
🚀 Why it was revolutionary?
✅ No checkout lines → Frictionless experience
✅ AI-driven inventory tracking → Smarter stock management
✅ Automated billing → No scanning needed
However, high costs, complexity, and consumer adoption barriers led Amazon to scale back the model.
🔍 Key Business Challenges & Lessons
1. Unsustainable Costs & Scaling Challenges
- AI Infrastructure Too Expensive: Required high-density sensors, cameras, and real-time processing, leading to excessive operating costs per store.
- Low Scalability: Unlike traditional retail models, each store required costly, customized AI calibration.
- Future Outlook: As AI costs decrease, the model may become viable, but today, ROI is insufficient.
AI-powered retail must balance innovation with cost efficiency to achieve sustainable scalability.
Amazon’s Just Walk Out (JWO) technology is now licensed to other retailers, including Whole Foods, Hudson stores, and smaller convenience outlets. Meanwhile, hybrid scan-and-go solutions have gained traction, blending AI efficiency with customer control.
For example, Walmart has implemented AI-driven checkout systems that have reportedly reduced inventory loss by 15%.
2. AI Complexity vs. Real-World Retail Operations
- Edge Cases Were Hard to Manage: Group shopping, misplaced items, and foot traffic created tracking inconsistencies.
- AI Misidentifications: Inaccuracies in product recognition led to billing errors and customer friction.
- Future Outlook: AI will improve, but human oversight and hybrid models may be necessary to enhance accuracy.
AI should be iteratively tested and adapted to real-world unpredictability before full-scale deployment.
AI-driven inventory tracking and smart shelving have been widely adopted in retail. Walmart, Kroger, and Sam’s Club now use AI-powered stock management systems to enhance supply chain efficiency and reduce losses.
3. Consumer Adoption Barriers
- Friction in User Experience: Requiring an Amazon app to enter stores deterred casual shoppers.
- Limited Product Selection: Amazon Go focused on convenience store goods, missing broader retail adoption.
- Future Outlook: As biometric payments and digital wallets improve, frictionless retail models will gain traction.
AI-driven retail should enhance, not disrupt, existing consumer behaviors.
4. Privacy & Ethical AI Concerns
- Surveillance Fears: AI-powered cameras raised concerns over data privacy and tracking transparency.
- Regulatory Risks: Future AI privacy laws could further restrict AI-driven monitoring.
- Future Outlook: AI in retail must balance convenience with ethical, transparent AI policies.
Companies must build AI compliance frameworks proactively to avoid backlash.
AI-driven loss prevention systems have been integrated into major retailers, blending computer vision with human monitoring to prevent fraud at self-checkout kiosks and high-theft areas.
🏗 The AI Retail Success Framework
Based on Amazon Go’s lessons, companies can adopt the following framework for AI-powered retail success:
1. Feasibility & Cost Optimization
- Conduct a cost-benefit analysis before implementing AI solutions.
- Prioritize scalable AI infrastructure that reduces long-term operational costs.
- Invest in AI models that offer incremental efficiency gains rather than full automation upfront.
2. Hybrid AI + Human Oversight
- Implement AI-assisted rather than fully AI-driven retail experiences.
- Introduce human oversight layers for edge cases AI struggles to handle.
- Ensure continuous AI monitoring and iterative improvements based on real-world interactions.
3. Customer-Centric AI Adoption
- Reduce consumer friction by integrating AI seamlessly into existing shopping behaviors.
- Avoid requiring additional steps (e.g., mandatory app downloads) that discourage adoption.
- Use personalization and convenience as key drivers, rather than pure automation.
4. Ethical & Regulatory Alignment
- Build AI models that adhere to privacy-first principles.
- Ensure compliance with data protection laws and emerging AI regulations.
- Educate consumers on how AI is used and offer opt-in transparency for data collection.
📊 AI Maturity Scale for Retail Transformation
Why Moving Too Fast Can Backfire
Many companies attempt to jump from a basic digital infrastructure to an advanced AI-driven model too quickly, often bypassing critical learning phases. This can lead to inefficient deployment, high costs, and operational disruption. The optimal AI adoption approach follows a structured maturity scale:
Stage | Characteristics | Strategic Focus |
Basic | Traditional retail with limited digital tools | Digitize operations, collect & clean data |
Data-Driven | Adoption of analytics & early automation | Build AI-ready infrastructure, test predictive analytics |
Assisted AI | AI supports decision-making but humans remain in control | Implement AI for supply chain, pricing, personalization |
Hybrid AI | AI automates some processes with human oversight | Optimize workflows, improve real-time analytics |
Precursor AI | Fully AI-driven model with automation-first approach | Ensure cost efficiency, AI ethics, consumer trust |
Attempting to leap directly into a Precursor AI model (like Amazon Go) without progressing through critical maturity stages significantly raises the risk of failure. Retailers must take a phased, strategic approach to AI adoption, ensuring that each stage— from basic digitization to full AI automation — is tested, optimized, and aligned with business objectives before advancing.
🚀 Not a failure, but a catalyst for change
Amazon Go’s failure was not about technology — it was about timing, strategy, and deployment. The vision was ahead of its time, but market readiness, regulatory alignment, and cost feasibility were not in place for sustainable success. While its execution faced obstacles, it will remain a a valuable experiment in AI-driven retail, laying the groundwork for future innovations.
The most successful AI transformations (e.g., Walmart, Tesla, McDonald's) evolve progressively, integrating AI in measured phases to fine-tune performance, control costs, and secure stakeholder buy-in.
- Checkout-free technology has evolved into hybrid models like scan-and-go and assisted checkout.
- AI-powered inventory tracking is now widely used in retail operations.
- Personalization has shifted toward AI-driven but privacy-conscious customer experiences.
- AI-driven loss prevention is increasingly implemented in self-checkout security.
The future of AI in retail isn’t cashierless — it’s frictionless. The question now isn't whether AI belongs in retail, it’s how businesses will implement it strategically.