What is Explainable AI (XAI)?
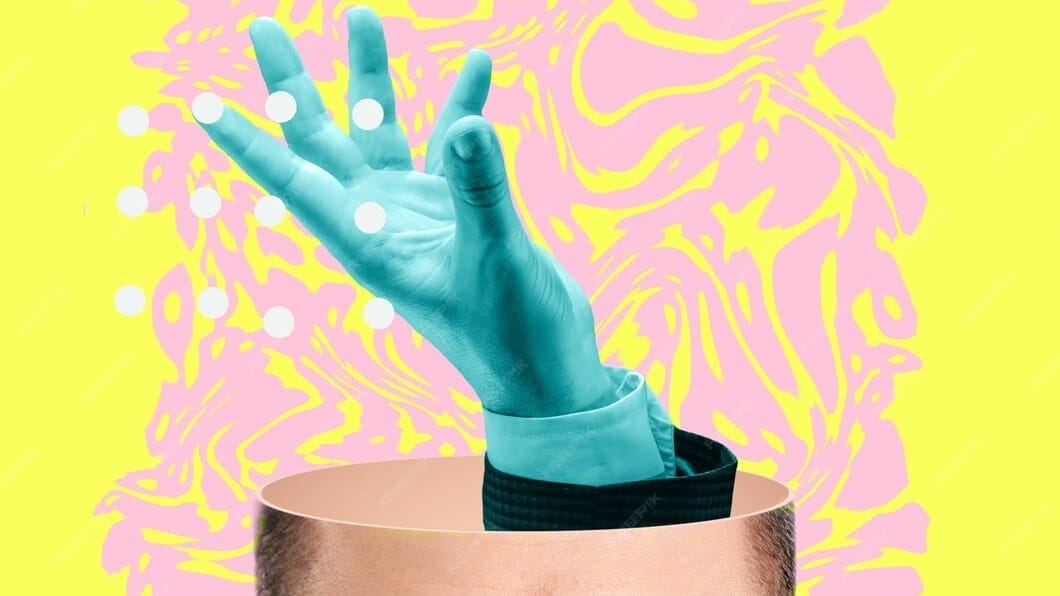
A Beginner's Guide to Understandable AI Systems 🤖
Often referred to as the “black box” problem, many AI systems provide outcomes without explaining how decisions are made. This can lead to distrust, bias, and unfair results. To support this, Explainable AI (XAI) is key.
🧠 What is Explainable AI?
Explainable AI (XAI) refers to AI systems designed to explain their decision-making processes in ways that humans can understand. Instead of being a "black box," XAI models provide insights into how a particular decision was reached, making it possible to identify biases, errors, or inconsistencies.
🌟 Why Does Explainable AI Matter?
As AI becomes more ingrained in areas like healthcare, finance, and recruitment, the need for accountability and trust in AI systems is more critical than ever. Here are a few key reasons why Explainable AI is important:
- Transparency: XAI allows users to see why an AI model made a decision, promoting trust.
- Fairness and Accountability: Without understanding AI decisions, it’s hard to hold anyone accountable for errors or biases. XAI helps ensure that AI operates fairly for all users.
- Regulatory Compliance: With increasing regulations like GDPR in Europe, businesses are required to provide explanations for AI-driven decisions. XAI supports compliance by making AI decisions traceable.
- Improved Decision-Making: Businesses can fine-tune AI models by understanding how decisions are made, improving overall performance and reliability.
⚙️ How Does Explainable AI Work?
Explainable AI uses a variety of methods to make AI decision-making more understandable. Some common techniques include:
- Feature Importance: This shows which factors influenced the AI’s decision the most. For example, in a loan approval, XAI can reveal that credit score and income were the deciding factors.
- Rule-Based Models: These systems explain decisions through a set of rules. For instance, a healthcare AI might explain a diagnosis based on specific symptoms.
- Visualizations: XAI often uses visual tools like heatmaps or charts to show how different inputs influenced the outcome, making it easier for users to interpret.
IBM Watson Health uses AI to assist doctors with diagnoses and treatment plans. By employing XAI, the system can explain which symptoms and data points (like patient history, test results, etc.) led to its recommendation. This transparency allows doctors to better understand and trust the AI's suggestions, while also ensuring that patients receive fair and accountable care. This approach has been especially useful in improving patient outcomes and addressing biases in medical data.
Here are a few other sectors where Explainable AI is making an impact:
Finance: In credit scoring, XAI helps banks explain why a loan was approved or denied, improving customer trust by revealing key factors like credit history and income.
Law Enforcement: XAI is used to explain predictive policing algorithms, ensuring recommendations are based on valid factors and not biased data.
Healthcare: XAI systems assist doctors by explaining the reasoning behind diagnoses, helping to identify the root causes and ensuring that decisions are fair and accurate.
🚧 Challenges of Explainable AI
Complex AI models, like deep learning, are often hard to interpret, making it difficult to provide clear explanations. Additionally, there’s often a trade-off between accuracy and explainability—more complex models tend to be more accurate but harder to understand.
🔮 The Future of Explainable AI
As AI continues to integrate into all aspects of society, the demand for explainable, transparent AI will only grow. Companies like Google, Microsoft, and IBM are investing heavily in XAI research and tools. Governments and regulators will likely continue to enforce stricter requirements for transparency in AI systems.
🏢 How Compagnies Can Leverage XAI
For compagnies incorporating AI into their products or services, XAI is crucial for building customer trust and ensuring responsible innovation. Here’s how startups can benefit from XAI:
- Trust and Transparency: By providing clear, understandable explanations for AI decisions, compagnies can foster trust with their users.
- Compliance: XAI helps compagnies stay ahead of regulatory requirements that mandate transparency in automated decision-making.
- Product Improvement: With insights from XAI, compagnies can identify potential errors or biases in their AI systems and make adjustments for more reliable results.
Explainable AI (XAI) is transforming the way we interact with AI systems, making them more transparent, fair, and trustworthy. By offering clear insights into the decision-making process, XAI not only improves user trust but also helps businesses comply with regulatory standards and ensure fair outcomes for all. As AI’s role in society continues to grow, Explainable AI will be key to fostering accountability and transparency in the digital world.