Understanding Algorithmic Bias: What It Is and Why It Matters
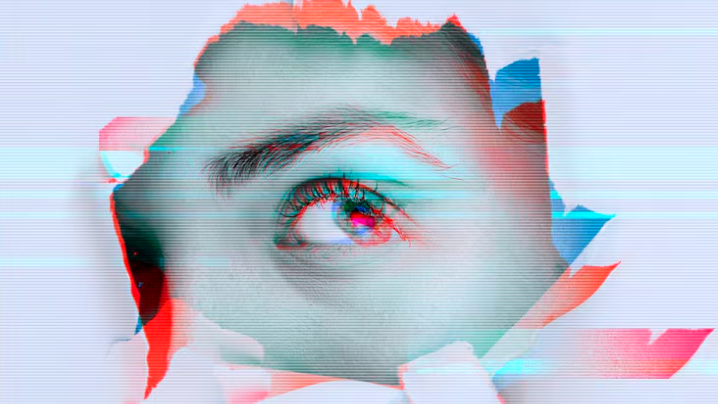
What Is Algorithmic Bias? 🤔
Algorithmic bias refers to systematic and unfair discrimination that can occur when algorithms make decisions based on flawed data or biased assumptions. This bias can lead to outcomes that reinforce existing inequalities, impacting marginalized groups disproportionately.
For instance, if an algorithm is trained on historical data that reflects societal biases, it may produce biased results, perpetuating discrimination. Recognizing and addressing algorithmic bias is vital to ensuring fairness and equity in technology.
Why Does Algorithmic Bias Matter? 🌍
- Social Justice: Algorithmic bias can reinforce stereotypes and perpetuate discrimination, affecting access to opportunities in education, employment, and beyond.
- Business Reputation: Companies that fail to address bias in their algorithms risk damaging their reputation and losing customer trust.
- Legal Implications: Failing to ensure fairness in algorithmic decisions can lead to legal challenges and regulatory scrutiny.
Real-World Examples of Algorithmic Bias 🚨
Case: Google developed a resume screening tool designed to help recruiters find qualified candidates more efficiently. However, it was found that the algorithm favored candidates from certain demographic backgrounds, leading to accusations of bias in the hiring process.
Impact: This situation emphasizes the importance of diverse training data and careful monitoring to prevent reinforcing existing biases in hiring practices.
Case: Microsoft’s Azure Face API was criticized for its inaccuracies in recognizing faces of people with darker skin tones. Studies showed that the technology misidentified women and people of color more frequently than white men.
Impact: This has raised ethical concerns about surveillance and law enforcement practices, as misidentification can lead to wrongful arrests and discrimination.
Case: ZestFinance, a financial technology company, developed an algorithm to assess creditworthiness using alternative data sources. However, the algorithm has faced scrutiny for potentially reinforcing biases present in the data, particularly against minority groups.
Impact: This raises questions about the fairness of using alternative data in credit scoring and the potential for perpetuating existing inequalities in financial access.
How to Mitigate Algorithmic Bias 🔧
Addressing algorithmic bias requires a multi-faceted approach:
- Diverse Data: Ensure that training data is diverse and representative of the population to avoid reinforcing stereotypes.
- Transparency: Companies should be transparent about their algorithms and how decisions are made. This builds trust and accountability.
- Regular Audits: Conduct regular audits of algorithms to identify and rectify biases. This should involve interdisciplinary teams that include ethicists, data scientists, and representatives from affected communities.
The Importance of Addressing Algorithmic Bias 🌟
Algorithmic bias is a pressing issue that can have significant social, legal, and business implications. By understanding what algorithmic bias is and why it matters, we can work toward creating fairer, more equitable systems in technology. As consumers, it’s essential to stay informed and advocate for ethical practices in the algorithms that influence our lives. Together, we can make a difference!