Algorithmic Accountability: Who Is Responsible?
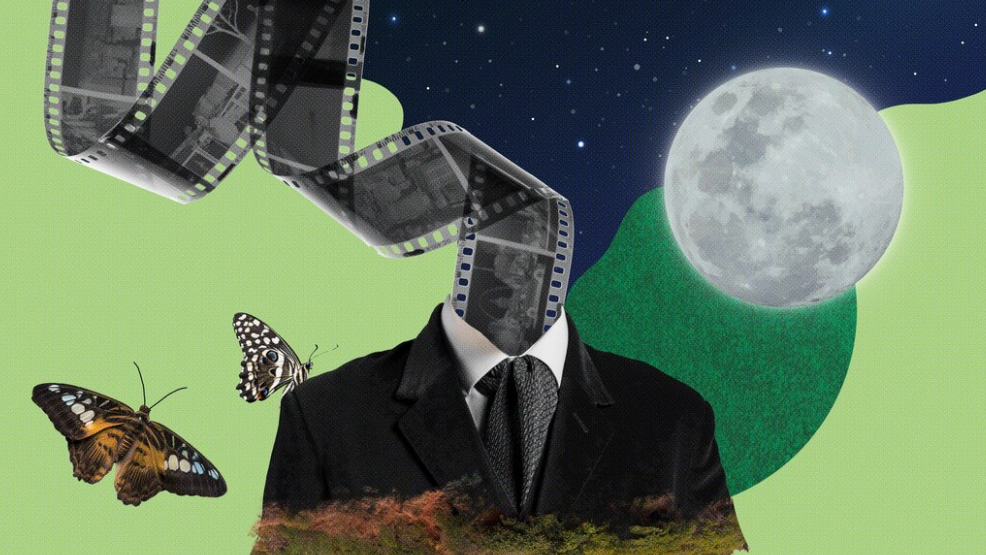
Driving decisions in hiring, healthcare, finance, and even in the justice system. As these technologies become more embedded in our daily lives, one important question arises: Who is responsible when an algorithm makes a mistake? This concept of algorithmic accountability is crucial to ensure fairness, transparency, and trust in our increasingly automated world.
đ What is Algorithmic Accountability?
Algorithmic accountability refers to the responsibility that developers, companies, and stakeholders have when designing, implementing, and deploying algorithms. When these systems make decisionsâespecially in high-stakes areas like hiring, credit scoring, or criminal justiceâensuring fairness and accuracy is critical.
If an algorithm produces biased or harmful results, who should be held accountable: the developers who designed it? The company that uses it? Or even the algorithm itself?
đ Why Algorithmic Accountability Matters?
- Fairness and Equity: Algorithms often reflect the biases present in their training data, which can lead to discriminatory outcomes, especially for marginalized groups.
- Trust: For people to trust AI systems, they need to know that companies are committed to ensuring that the algorithms are fair and transparent.
- Legal and Regulatory Compliance: With increasing regulations around data privacy and AI, such as the EU's General Data Protection Regulation (GDPR), companies are legally required to ensure accountability in their AI systems.
đ§ Who Is Responsible?
Algorithmic accountability involves multiple players:
- Developers: The engineers and data scientists who design algorithms are responsible for ensuring that the models are trained on unbiased, diverse datasets and perform fairly across different demographics.
- Companies: The organizations that deploy algorithms bear responsibility for their impact. They need to ensure that AI models align with ethical guidelines, are regularly tested, and are transparent about how decisions are made.
- End Users: Even users of algorithmic tools, like hiring managers or financial institutions, share some responsibility. They should understand how these systems work and be mindful of relying too heavily on automated decisions.
đ¨ Real-World Examples of Algorithmic Accountability
Case: X came under fire in 2020 when users discovered that its image-cropping algorithm was biased. The AI tool consistently cropped out Black faces, prioritizing white faces in preview images.
Accountability: X apologized and quickly began reworking the algorithm to improve fairness. However, this case highlights the need for companies to test their algorithms thoroughly before deployment, especially in systems that affect how people are represented online.
Case: In 2019, Apple Card, which is backed by Goldman Sachs, faced allegations of gender bias when reports surfaced that women were being given lower credit limits than men, even when they had higher credit scores. The AI system determining creditworthiness seemed to favor men.
Accountability: Goldman Sachs responded by investigating the issue, though the lack of transparency in how credit decisions were made made it difficult to pinpoint the source of bias. This case illustrates the importance of providing clear explanations and transparency in algorithmic decisions, especially in financial services.
đ§ How to Ensure Algorithmic Accountability?
To avoid the pitfalls of biased or harmful algorithms, companies must take responsibility by adopting a series of best practices:
- Regular Audits: Conduct ongoing audits of AI systems to identify any biases and correct them. These audits should involve diverse teams that include ethicists, data scientists, and stakeholders from different backgrounds.
- Transparency: Ensure that algorithms are explainable and that users understand how decisions are made. Black-box algorithmsâwhere the decision-making process is opaqueâerode trust.
- Inclusive Data: Use training data that reflects a wide range of demographics and experiences to reduce bias and improve fairness.
- Human Oversight: Algorithms should not be left to make important decisions in isolation. Human oversight is essential to verify that the results are fair and align with ethical standards.
đ Key Takeaways: Sharing the Responsibility for Ethical Algorithms
Algorithmic accountability is not about assigning blame to a single party. Instead, itâs about sharing responsibility among developers, companies, and end users to ensure that algorithms are fair, transparent, and responsible. As AI continues to shape our world, fostering trust and accountability will be key to its ethical and equitable use.
Businesses that take accountability seriouslyâthrough transparency, audits, and diverse data practicesâare more likely to maintain consumer trust and avoid regulatory pitfalls.